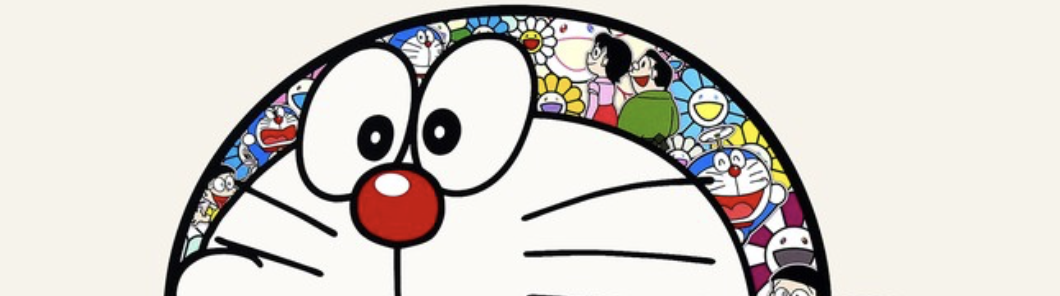
What I have learnt from being a Data Scientist Intern
Time flies. My ten-weeks summer internship just ended last week, and now may be a good time for me to reflect on the journey and summarize what I have learned from this valuable experience.
1. communication & communication & communication
Communication is now a totally different thing than what I thought it was before the internship. Before, communication meant either a casual chat with friends or a professional presentation of the research work. After the internship, I realized that communication is about advocating for oneself strategically.
Throughout the internship, I met and greeted with on average two or three new colleagues every week, I learned to develop a dynamic communication style when talking with people from various backgrounds and with distinct personalities. I had weekly meetings with my supervisors, and I learned to craft message based on the other person’s position and interests.
I am proud of myself being aware of those improvement opportunities, but still I feel there were many moments where I could have advocated more for myself. For instances, when being asked a general question, I often just gave a direct and concise answer, but it would be better if I could offer more context and individual opinions. Also when hearing feedback from the supervisors about my performance, I would reflect on the comments and try to improve accordingly. Those feedback are valuable, but it’s equally important that one should be proactive on giving feedback on the feedback — advocate the things you regard as strengths but haven’t been noticed by your supervisor yet, explain more about the things deemed as weaknesses by others but on which you actually have different perspectives. It’s not bragging about oneself, it’s building reputation and getting recognized.
I used to feel self-conscious on advocating myself by saying than doing. Now I would say I am ready to talk loud about myself 🥁.
2. learn to make a good judgement call
I would say one thing that distinguishes the academic research work and industry production work is that, research puts more focus on having a thorough and rigorous methodology first, then the results are generated and evaluated. However, the industry puts more focus on getting a result first, then spends time on validating and improving the methodology implemented. It’s very understandable because they are driven by different goals, one is innovation and the other one is profit. As a result, a hurdle that people diverting from academic to industry often stumble over is that they tend to get into the weeds and lose the whole picture, thus less productive. Therefore, it’s very critical to know when to make a good judgement call.
When I started my internship, I had to learn a new statistical model that I hadn’t used before, and there was an entire book talking about the theory and its implementations, which would take me weeks to finish reading all the materials. But I only had 10 weeks working on the project and there were lots of other tasks need to be taken care of at the same time. So it was a moment where I had to make a good judgement call. I knew I didn’t have to be an expert on using this model but what should I learn first? How much should I learn so that I can apply the model in a correct way? There was not a quick solution to this, so I started with experimenting the models to first learn how to use them, and I set aside ~1hour every day to look at the tutorial that was most concerned to the question I had at that moment, and spent the rest of time working on other parts of the project. By doing this for 5 or 6 weeks, I finally got a good understanding of the statistical model, meanwhile, progress was made on the project as scheduled.
Throughout the internship, there were may other moments where I was consciously aware of that a judgement call needs to be made. Such awareness is the second thing I learned from the internship and I will use that on my future research work to stay productive.
3. make good use of your available tool-kit
Before I started the internship, I was a bit concerned that though I was proficient in R programming and statistical models, I lacked experience of using machine learning or deep learning models that are prevailing and favored in the data scientist works. I was trapped in the idea that being able to use a “fancy” and innovative model is better than using an “old” but well-established model, so I often found myself asking people that “what new models you are using?”.
Then during my internship, I talked to a colleague who has since inspired me to look at the concern differently. He said his modeling philosophy is that, using the least tools to solve the most problems. I couldn’t agree more with that. I can never learn and be good at everything, but I can learn to make full use of the tools I already have.
4. be open minded about career possibilities
One of my favorite internship stories is from the CEO of the company. In a celebration event with all the interns, the CEO spoke about how he founded the company at the beginning. He was initially working as a strategic consultant, in one afternoon, he talked with a friend who worked in the banking industry, after that he immediately saw a big business opportunity of issuing credit card in a way that different from the traditional banks. He came up with the idea in one afternoon, took another five years to have the company founded, and the company went public seven years later. I like the entrepreneur spirit demonstrated in the story, and the CEO being adventurous and determined to make a good idea come true.
I was also impressed by the diverse backgrounds and agility of people who are working as the data scientists in the company. There are people coming from astronomy, physics, mathematics, geophysics, economy, consulting, etc. In addition, the company offered plenty of trainings and workshops to help data scientists learn the business strategies closely related to the modeling works, which I enjoyed a lot.
All those things motivate me to think that while becoming good at a specific job, I should also be open minded to other career possibilities, cultivating the versatile skills that can be applied to different occasions. After all, I have the heart of an adventurer 🐌.
5. do not apologize for your own success, but recognize and applaud others
The words above is a quote from a speaker in a meeting I attended during the internship. It was an enlightening moment the first time I saw it. That I like the quote does not mean I am already successful, in fact, it clears the could over the jealousy and defeated feeling I had every time when I compared my performance with others. The way I interpret it is that, do your best to achieve YOUR goals and be aware and grateful to others that have helped along the way.
After writing down this article, I realized I actually learned more soft skills than hard skills from this internship as a data scientist. I am grateful for this valuable experience and I am positive that those new acquired skills will help me thrive in any jobs regardless of whether it’s a data scientist or not.